We strive to create an inclusive, collaborative and connected culture that fosters cutting-edge basic, translational, education, and health outcomes research. Our research programs investigate the underlying mechanisms of disease, translate discoveries in the laboratory to clinical trials, and offer experimental options with the goal of improving patient care. Our research funding portfolio exceeds $18 million annually with a large percentage from the National Institutes of Health.
Our Researchers & Labs >>
Latest Research News
Wisconsin Surgery Research Roundup: May 2025
Wisconsin Department of Surgery members engage in remarkable research that yields many impactful publications every month. We’re highlighting several of these publications monthly to showcase the diversity of research in the department; see selections from …
Chiu Awarded Grant to Reduce Disparities in Primary Hyperparathyroidism Treatment
Primary hyperparathyroidism (PHPT) is a condition in which the parathyroid glands produce too much parathyroid hormone. If left untreated, this condition significantly increases the risk of bone fractures, kidney stones, and cardiovascular events. While surgical …
Postdoc in WiSLiM Lab Receives Funding from UW Distinguished Research Fellows Program
Reji Babygirija, PhD, a postdoctoral scientist in the Wisconsin Surgical Laboratory in Metabolism (WiSLiM), has been awarded a UW Distinguished Research Fellowship. This fellowship program was established by the Office of the Vice Chancellor for …
WiSLiM Lab Receives NIH Funding to Examine How Bariatric Surgery Affects Alzheimer’s Disease Risk
Obesity and type 2 diabetes can increase the risk of Alzheimer’s disease. Research in animal models has shown that dietary interventions like calorie or protein restriction can improve metabolic health as well as slow or …
Wisconsin Surgery Research Roundup: April 2025
Wisconsin Department of Surgery members engage in remarkable research that yields many impactful publications every month. We’re highlighting several of these publications monthly to showcase the diversity of research in the department; see selections from …
OHNS Faculty Member Awarded Prestigious NIH Career Development Award
Division of Otolaryngology-Head and Neck Surgery Assistant Professor Ruth Davis, MD, was recently awarded a 5-year, $711,000 career development award from the National Institute on Deafness and Other Communication Disorders. NIH career development awards provide …
Brown Lab Awarded Grant to Study Potential New Treatment for Type 1 Diabetes
The lab of Division of Transplantation Assistant Professor Matthew E. Brown, PhD, is hyper-focused on creating hypoimmune stem cells – cells that can evade recognition and destruction by the human immune system. Why? Because stem …
Wisconsin Surgery Research Roundup: March 2025
Wisconsin Department of Surgery members engage in remarkable research that yields many impactful publications every month. We’re highlighting several of these publications monthly to showcase the diversity of research in the department; see selections from …
Dr. Sean Ronnekleiv-Kelly Receives Grant to Study the Link between Three Rare Biliary Cancers
Division of Surgical Oncology Associate Professor Sean Ronnekleiv-Kelly, MD, has devoted much of his scientific career to the study of fibrolamellar cancer, a rare but deadly form of liver cancer that affects young adults. So …
Dr. Carrie Thiessen Awarded Prestigious NIH Career Development Award
Division of Transplantation Assistant Professor Carrie Thiessen, MD, PhD, was recently awarded a 5-year, $956,000 career development award from the National Institute of Diabetes and Digestive and Kidney Diseases. NIH career development awards provide early-stage …
Zafar Awarded Grant to Explore Social Determinants of Health in GI Cancer Surgery Outcomes
Gastrointestinal (GI) cancers – such as pancreatic, colorectal, and liver cancer – often require surgical treatment. Because these procedures are complex, complications can happen. “Cancer surgery complications are associated with a variety of factors, including …
Cho Lab Receives Funding from the Draper Technology Innovation Fund
Division of Plastic Surgery Assistant Professor Daniel Cho, MD, PhD, specializes in the treatment of infants and children with birth defects that affect the head and skull. One such defect, craniosynostosis, is a serious condition …
Doctoral Student in Odorico Lab Receives Prestigious Graduate Training Award from UW Stem Cell & Regenerative Medicine Center
Second-year Cellular and Molecular Biology PhD student Caterra Leavens’ mission to develop a cure for Type 1 diabetes is personal; as a Type 1 diabetic herself, she is very familiar with the complexities of the …
Wisconsin Surgery Research Roundup: February 2025
Wisconsin Department of Surgery members engage in remarkable research that yields many impactful publications every month. We’re highlighting several of these publications monthly to showcase the diversity of research in the department; see selections from …
Surgery and Engineering Researchers Team Up to Enhance Wound Healing
Chronic wounds – wounds that haven’t started to heal after several weeks of treatment – affect over 6.5 million people in the U.S., are a threat to patients’ health and quality of life, and have …
- More Research posts
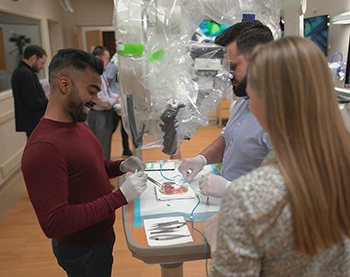
Research Leadership
Luke Funk, MD, MPH
Vice Chair of Research
Angela Gibson, MD, PhD
Vice Chair of Research
JoAnne Vaccaro
Director of Research Operations
Clinical Trials Core
Provides coordinator and regulatory support for all types of human subjects research including sponsored clinical trials, survery studies, registries, and chart reviews.
UW Humanized Mouse Core
The Humanized Mouse Core at the University of Wisconsin–Madison provides investigators with a variety of humanized mouse models for their individual research needs.
Statistical Analysis and Research Programming (STARP) Core
Provides faculty, researchers, and residents/fellows/students with comprehensive statistical and research programming support for their research activities including study design, grant application, abstract/manuscript review, statistical analysis, programming and results interpretation.
WiSOR Qualitative Core
The WiSOR Qualitative Core offers faculty and staff consultation, support, and expertise on study design, methodology, data collection and analysis, and interpretation of results. It also offers transcription services.